Pavement Crack Segmentation Paper
2020 年 1 月收集的关于 Pavement Crack Segmentation 的最新论文,pdf 地址换上 arXiv 网址即可。
目录
1.A Deep Neural Networks Approach for Pixel-Level Runway Pavement Crack Segmentation Using Drone-Captured Images
arXiv:2001.03257 [pdf] cs.CV eess.IV
Authors: Liming Jiang, Yuanchang Xie, Tianzhu Ren
Abstract: Pavement conditions are a critical aspect of asset management and directly affect safety. This study introduces a deep neural network method called U-Net for pavement crack segmentation based on drone-captured images to reduce the cost and time needed for airport runway inspection. The proposed approach can also be used for highway pavement conditions assessment during off-peak periods when there are few vehicles on the road. In this study, runway pavement images are collected using drone at various heights from the Fitchburg Municipal Airport (FMA) in Massachusetts to evaluate their quality and applicability for crack segmentation, from which an optimal height is determined. Drone images captured at the optimal height are then used to evaluate the crack segmentation performance of the U-Net model. Deep learning methods typically require a huge set of annotated training datasets for model development, which can be a major obstacle for their applications. An online annotated pavement image dataset is used together with the FMA data to train the U-Net model. The results show that U-Net performs well on the FMA testing data even with limited FMA training images, suggesting that it has good generalization ability and great potential to be used for both airport runways and highway pavements.
Submitted 9 January, 2020; originally announced January 2020.
Comments: 13 pages, 5 figures
2.Automated Pavement Crack Segmentation Using Fully Convolutional U-Net with a Pretrained ResNet-34 Encoder
arXiv:2001.01912 [pdf] cs.CV
Authors: Stephen L. H. Lau, Xin Wang, Xu Yang, Edwin K. P. Chong
Abstract: Automated pavement crack segmentation is a challenging task because of inherent irregular patterns and lighting conditions, in addition to the presence of noise in images. Conventional approaches require a substantial amount of feature engineering to differentiate crack regions from non-affected regions. In this paper, we propose a deep learning technique based on a convolutional neural network to perform segmentation tasks on pavement crack images. Our approach requires minimal feature engineering compared to other machine learning techniques. The proposed neural network architecture is a modified U-Net in which the encoder is replaced with a pretrained ResNet-34 network. To minimize the dice coefficient loss function, we optimize the parameters in the neural network by using an adaptive moment optimizer called AdamW. Additionally, we use a systematic method to find the optimum learning rate instead of doing parametric sweeps. We used a “one-cycle” training schedule based on cyclical learning rates to speed up the convergence. We evaluated the performance of our convolutional neural network on CFD, a pavement crack image dataset. Our method achieved an F1 score of about 96%. This is the best performance among all other algorithms tested on this dataset, outperforming the previous best method by a 1.7% margin.
Submitted 10 January, 2020; v1 submitted 7 January, 2020; originally announced January 2020.
Comments: 9 pages, 6 figures
3. CrackGAN: A Labor-Light Crack Detection Approach Using Industrial Pavement Images Based on Generative Adversarial Learning
arXiv:1909.08216 [pdf, other] cs.CV cs.LG eess.IV
Authors: Kaige Zhang, Yingtao Zhang, Heng-Da Cheng
Abstract: Fully convolutional network is a powerful tool for per-pixel semantic segmentation/detection. However, it is problematic when coping with crack detection using industrial pavement images: the network may easily “converge” to the status that treats all the pixels as background (BG) and still achieves a very good loss, named “All Black” phenomenon, due to the data imbalance and the unavailability of accurate ground truths (GTs). To tackle this problem, we introduce crack-patch-only (CPO) supervision and generative adversarial learning for end-to-end training, which forces the network to always produce crack-GT images while reserves both crack and BG-image translation abilities by feeding a larger-size crack image into an asymmetric U-shape generator to overcome the “All Black” issue. The proposed approach is validated using four crack datasets; and achieves state-of-the-art performance comparing with that of the recently published works in efficiency and accuracy.
Submitted 18 September, 2019; originally announced September 2019.
4. A Cost Effective Solution for Road Crack Inspection using Cameras and Deep Neural Networks
arXiv:1907.06014 [pdf] cs.CV cs.LG eess.IV
Authors: Qipei Mei, Mustafa Gül
Abstract: Automatic crack detection on pavement surfaces is an important research field in the scope of developing an intelligent transportation infrastructure system. In this paper, a cost effective solution for road crack inspection by mounting commercial grade sport camera, GoPro, on the rear of the moving vehicle is introduced. Also, a novel method called ConnCrack combining conditional Wasserstein generative adversarial network and connectivity maps is proposed for road crack detection. In this method, a 121-layer densely connected neural network with deconvolution layers for multi-level feature fusion is used as generator, and a 5-layer fully convolutional network is used as discriminator. To overcome the scattered output issue related to deconvolution layers, connectivity maps are introduced to represent the crack information within the proposed ConnCrack. The proposed method is tested on a publicly available dataset as well our collected data. The results show that the proposed method achieves state-of-the-art performance compared with other existing methods in terms of precision, recall and F1 score.
Submitted 22 October, 2019; v1 submitted 13 July, 2019; originally announced July 2019.
5.FPCNet: Fast Pavement Crack Detection Network Based on Encoder-Decoder Architecture
arXiv:1907.02248 [pdf, other] cs.CV
Authors: Wenjun Liu, Yuchun Huang, Ying Li, Qi Chen
Abstract: Timely, accurate and automatic detection of pavement cracks is necessary for making cost-effective decisions concerning road maintenance. Conventional crack detection algorithms focus on the design of single or multiple crack features and classifiers. However, complicated topological structures, varying degrees of damage and oil stains make the design of crack features difficult. In addition, the contextual information around a crack is not investigated extensively in the design process. Accordingly, these design features have limited discriminative adaptability and cannot fuse effectively with the classifiers. To solve these problems, this paper proposes a deep learning network for pavement crack detection. Using the Encoder-Decoder structure, crack characteristics with multiple contexts are automatically learned, and end-to-end crack detection is achieved. Specifically, we first propose the Multi-Dilation (MD) module, which can synthesize the crack features of multiple context sizes via dilated convolution with multiple rates. The crack MD features obtained in this module can describe cracks of different widths and topologies. Next, we propose the SE-Upsampling (SEU) module, which uses the Squeeze-and-Excitation learning operation to optimize the MD features. Finally, the above two modules are integrated to develop the fast crack detection network, namely, FPCNet. This network continuously optimizes the MD features step-by-step to realize fast pixel-level crack detection. Experiments are conducted on challenging public CFD datasets and G45 crack datasets involving various crack types under different shooting conditions. The distinct performance and speed improvements over all the datasets demonstrate that the proposed method outperforms other state-of-the-art crack detection methods.
Submitted 4 July, 2019; originally announced July 2019.
6.Feature Pyramid and Hierarchical Boosting Network for Pavement Crack Detection
arXiv:1901.06340 [pdf, other] cs.CV
Authors: Fan Yang, Lei Zhang, Sijia Yu, Danil Prokhorov, Xue Mei, Haibin Ling
Abstract: Pavement crack detection is a critical task for insuring road safety. Manual crack detection is extremely time-consuming. Therefore, an automatic road crack detection method is required to boost this progress. However, it remains a challenging task due to the intensity inhomogeneity of cracks and complexity of the background, e.g., the low contrast with surrounding pavements and possible shadows with similar intensity. Inspired by recent advances of deep learning in computer vision, we propose a novel network architecture, named Feature Pyramid and Hierarchical Boosting Network (FPHBN), for pavement crack detection. The proposed network integrates semantic information to low-level features for crack detection in a feature pyramid way. And, it balances the contribution of both easy and hard samples to loss by nested sample reweighting in a hierarchical way. To demonstrate the superiority and generality of the proposed method, we evaluate the proposed method on five crack datasets and compare it with state-of-the-art crack detection, edge detection, semantic segmentation methods. Extensive experiments show that the proposed method outperforms these state-of-the-art methods in terms of accuracy and generality.
Submitted 24 January, 2019; v1 submitted 18 January, 2019; originally announced January 2019.
7.Brain-inspired robust delineation operator
arXiv:1811.10240 [pdf, other] cs.CV
Authors: Nicola Strisciuglio, George Azzopardi, Nicolai Petkov
Abstract: In this paper we present a novel filter, based on the existing COSFIRE filter, for the delineation of patterns of interest. It includes a mechanism of push-pull inhibition that improves robustness to noise in terms of spurious texture. Push-pull inhibition is a phenomenon that is observed in neurons in area V1 of the visual cortex, which suppresses the response of certain simple cells for stimuli of preferred orientation but of non-preferred contrast. This type of inhibition allows for sharper detection of the patterns of interest and improves the quality of delineation especially in images with spurious texture. We performed experiments on images from different applications, namely the detection of rose stems for automatic gardening, the delineation of cracks in pavements and road surfaces, and the segmentation of blood vessels in retinal images. Push-pull inhibition helped to improve results considerably in all applications.
Submitted 26 November, 2018; originally announced November 2018.
Comments: Accepted at Brain-driven Computer Vision workshop at ECCV 2018
8.Automatic Pavement Crack Detection Based on Structured Prediction with the Convolutional Neural Network
arXiv:1802.02208 [pdf, other] cs.CV
Authors: Zhun Fan, Yuming Wu, Jiewei Lu, Wenji Li
Abstract: Automated pavement crack detection is a challenging task that has been researched for decades due to the complicated pavement conditions in real world. In this paper, a supervised method based on deep learning is proposed, which has the capability of dealing with different pavement conditions. Specifically, a convolutional neural network (CNN) is used to learn the structure of the cracks from raw images, without any preprocessing. Small patches are extracted from crack images as inputs to generate a large training database, a CNN is trained and crack detection is modeled as a multi-label classification problem. Typically, crack pixels are much fewer than non-crack pixels. To deal with the problem with severely imbalanced data, a strategy with modifying the ratio of positive to negative samples is proposed. The method is tested on two public databases and compared with five existing methods. Experimental results show that it outperforms the other methods.
Submitted 1 February, 2018; originally announced February 2018.
9.Road Crack Detection Using Deep Convolutional Neural Network and Adaptive Thresholding
arXiv:1904.08582 [pdf, other] cs.CV cs.LG eess.IV
Authors: Rui Fan, Mohammud Junaid Bocus, Yilong Zhu, Jianhao Jiao, Li Wang, Fulong Ma, Shanshan Cheng, Ming Liu
Abstract: Crack is one of the most common road distresses which may pose road safety hazards. Generally, crack detection is performed by either certified inspectors or structural engineers. This task is, however, time-consuming, subjective and labor-intensive. In this paper, we propose a novel road crack detection algorithm based on deep learning and adaptive image segmentation. Firstly, a deep convolutional neural network is trained to determine whether an image contains cracks or not. The images containing cracks are then smoothed using bilateral filtering, which greatly minimizes the number of noisy pixels. Finally, we utilize an adaptive thresholding method to extract the cracks from road surface. The experimental results illustrate that our network can classify images with an accuracy of 99.92%, and the cracks can be successfully extracted from the images using our proposed thresholding algorithm. △ Less
Submitted 17 April, 2019; originally announced April 2019.
Comments: 6 pages, 8 figures, 2019 IEEE Intelligent Vehicles Symposium
10.Road Damage Detection Using Deep Neural Networks with Images Captured Through a Smartphone
arXiv:1801.09454 [pdf, other] cs.CV cs.CY
Authors: Hiroya Maeda, Yoshihide Sekimoto, Toshikazu Seto, Takehiro Kashiyama, Hiroshi Omata
Abstract: Research on damage detection of road surfaces using image processing techniques has been actively conducted, achieving considerably high detection accuracies. Many studies only focus on the detection of the presence or absence of damage. However, in a real-world scenario, when the road managers from a governing body need to repair such damage, they need to clearly understand the type of damage in order to take effective action. In addition, in many of these previous studies, the researchers acquire their own data using different methods. Hence, there is no uniform road damage dataset available openly, leading to the absence of a benchmark for road damage detection. This study makes three contributions to address these issues. First, to the best of our knowledge, for the first time, a large-scale road damage dataset is prepared. This dataset is composed of 9,053 road damage images captured with a smartphone installed on a car, with 15,435 instances of road surface damage included in these road images. In order to generate this dataset, we cooperated with 7 municipalities in Japan and acquired road images for more than 40 hours. These images were captured in a wide variety of weather and illuminance conditions. In each image, we annotated the bounding box representing the location and type of damage. Next, we used a state-of-the-art object detection method using convolutional neural networks to train the damage detection model with our dataset, and compared the accuracy and runtime speed on both, using a GPU server and a smartphone. Finally, we demonstrate that the type of damage can be classified into eight types with high accuracy by applying the proposed object detection method. The road damage dataset, our experimental results, and the developed smartphone application used in this study are publicly available (https://github.com/sekilab/RoadDamageDetector/).
Submitted 1 February, 2018; v1 submitted 29 January, 2018; originally announced January 2018.
Comments: 14 pages, 7 figures
本文作者 : HeoLis
原文链接 : https://ishero.net/Pavement%20Crack%20Segmentation%20Paper.html
版权声明 : 本博客所有文章除特别声明外,均采用 CC BY-NC-SA 4.0 许可协议。转载请注明出处!
学习、记录、分享、获得
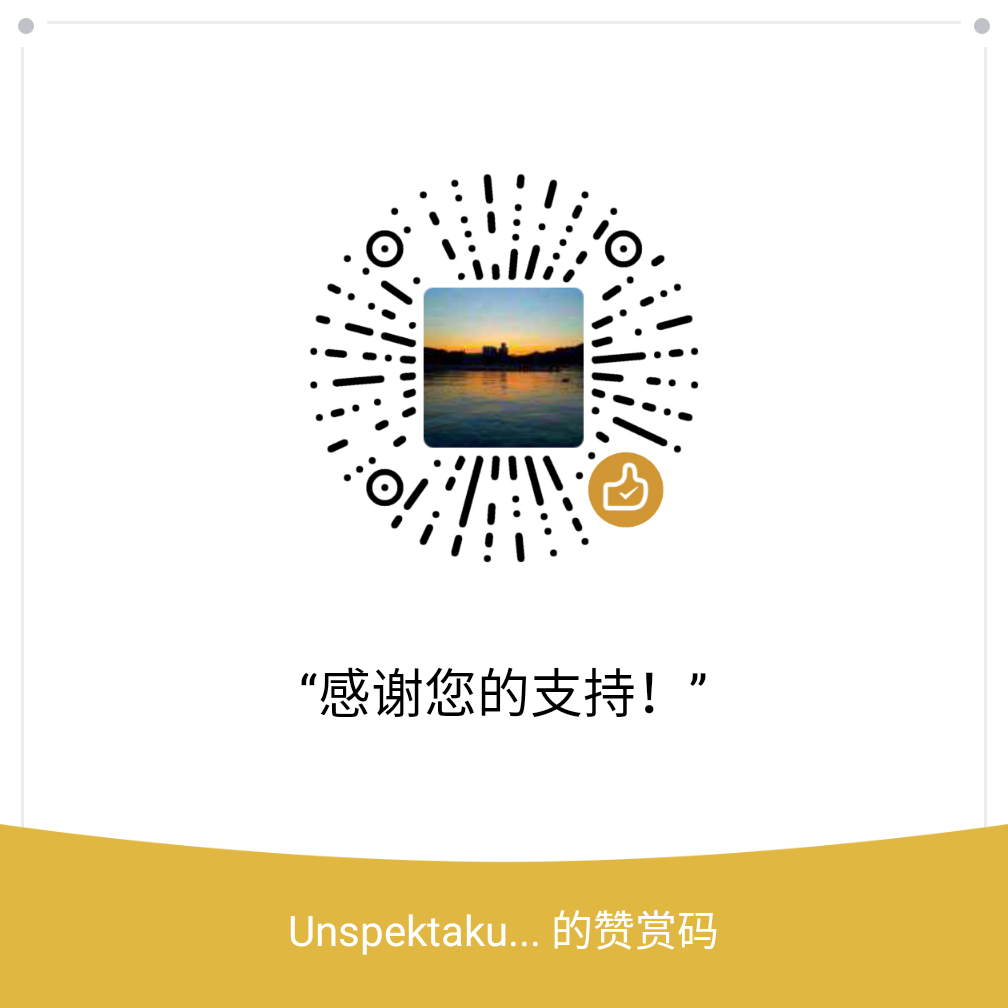